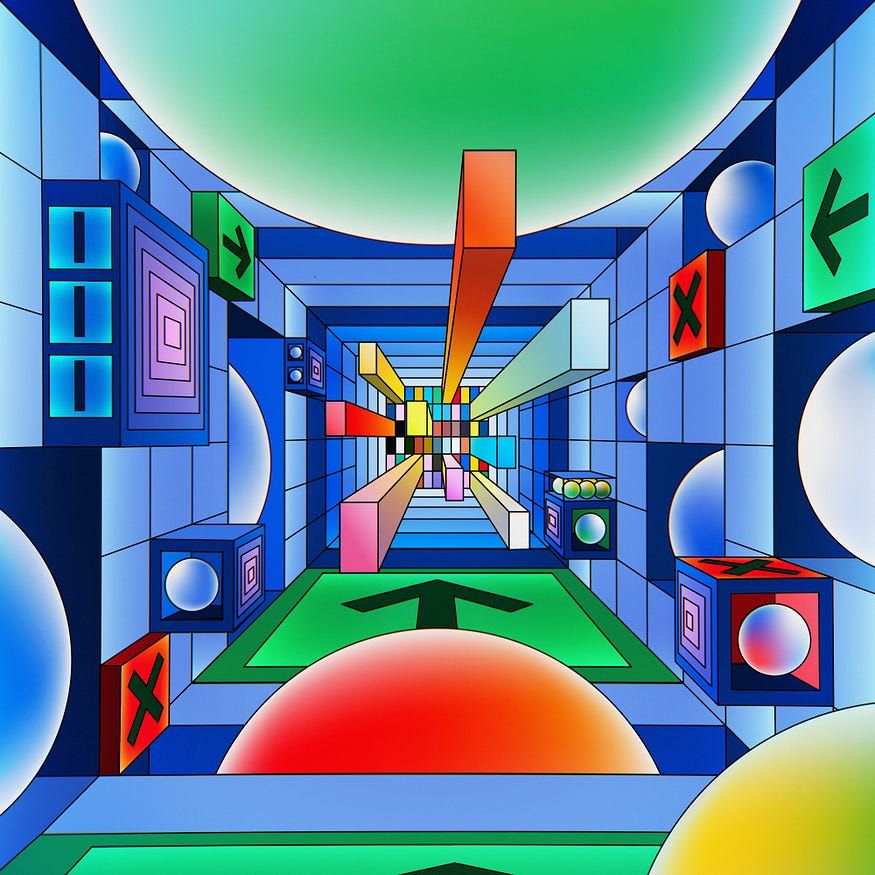
If you haven't already, you might have heard about prompt engineering. Essentially, it is the art of effectively communicating with an AI to achieve your desired results.
However, many people struggle with crafting good prompts.
Nevertheless, this skill is becoming increasingly important because the quality of the input directly affects the quality of the output.
Here are some essential techniques to master in the art of prompting:
I will refer to a language model as 'LM'.
Examples of language models include ChatGPT by OpenAI and Claude by AnthropicAI.
1. Persona/role prompting
Assign a specific role to the AI.
For example, you can say, "You are an expert in X. You have been helping people with Y for 20 years. Your task is to provide the best advice about X. Please reply with 'got it' if you understand."
An additional powerful component to include is:
"You must always ask clarifying questions before providing an answer, so you can fully understand what the questioner is looking for."
I will explain why this is crucial shortly.
2. CoT (Chain of Thought)
CoT is used to instruct the LM to explain its reasoning.
For instance:
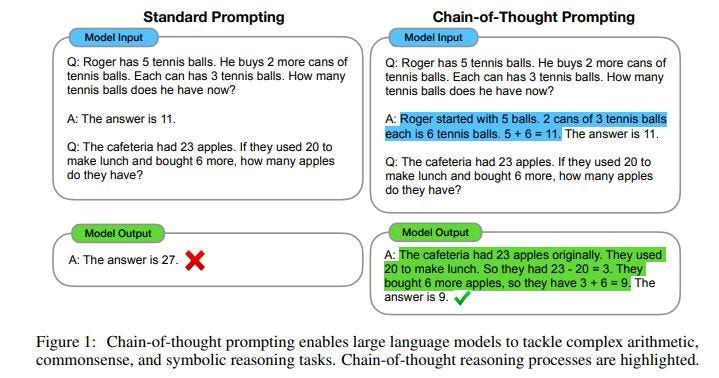
3. Zero-shot-CoT
Zero-shot refers to using a model to make predictions without giving it additional training within the prompt.
I will touch on few-shot in a moment.
Typically, CoT is more effective than Zero-shot-CoT.
For example:
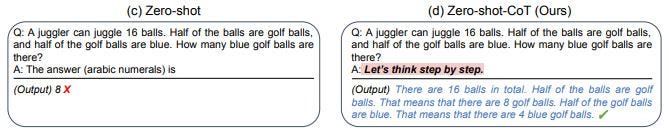
4. Few-shot (and few-shot-CoT)
In few-shot learning, the LM is given a few examples in the prompt to help it quickly adapt to new examples.
For example:
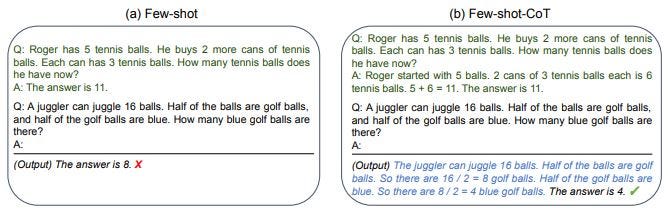
5. Knowledge generation
You can prompt an LM to generate knowledge related to a specific question.
This can be useful for generating knowledge prompts (as explained further below).
For example:
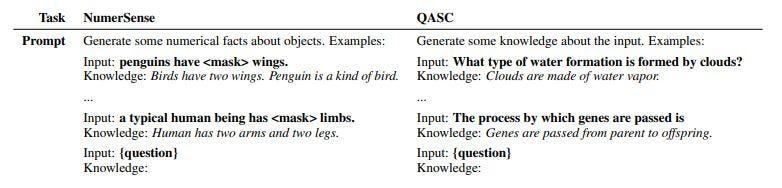
6. Generated knowledge
Once you have generated knowledge, you can feed that information into a new prompt and ask questions related to that knowledge.
Questions of this nature are called 'knowledge augmented' questions.
7. Self-consistency
This technique is used to create multiple reasoning paths (chains of thought).
The final answer is determined by the majority consensus.
For example:
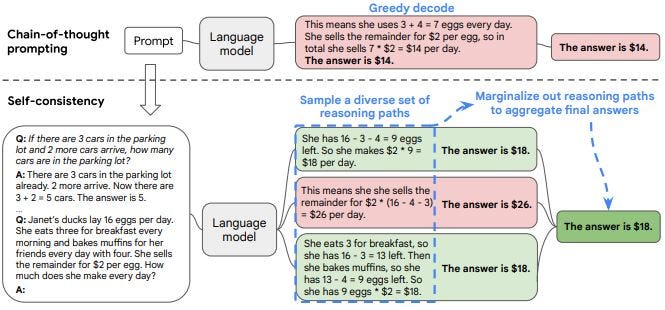
8. LtM (Least to Most)
LtM stands for 'Least to Most'.
This technique builds upon CoT and involves breaking down a problem into subproblems and solving them individually.
For example:
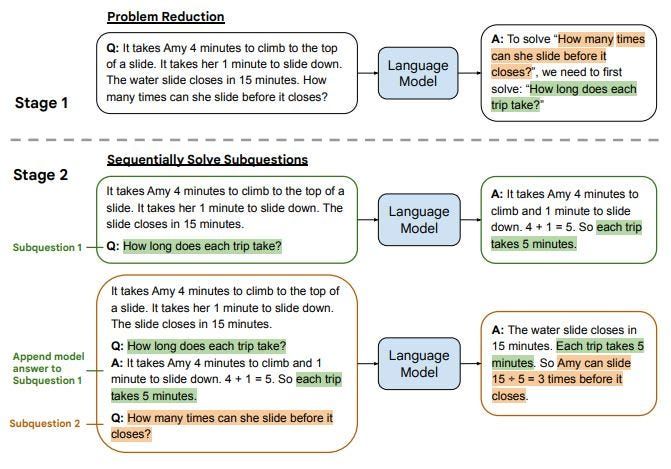